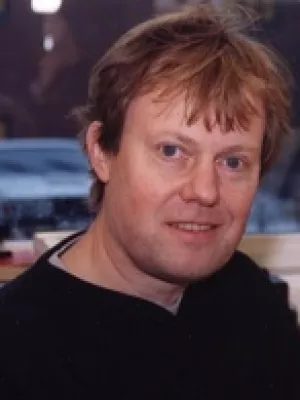
Åke Borg
Principal investigator
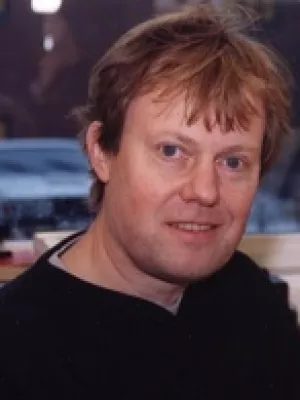
A deep learning system accurately classifies primary and metastatic cancers using passenger mutation patterns
Author
Other contributions
- Åke Borg
- Markus Ringnér
- Johan Staaf
Summary, in English
In cancer, the primary tumour's organ of origin and histopathology are the strongest determinants of its clinical behaviour, but in 3% of cases a patient presents with a metastatic tumour and no obvious primary. Here, as part of the ICGC/TCGA Pan-Cancer Analysis of Whole Genomes (PCAWG) Consortium, we train a deep learning classifier to predict cancer type based on patterns of somatic passenger mutations detected in whole genome sequencing (WGS) of 2606 tumours representing 24 common cancer types produced by the PCAWG Consortium. Our classifier achieves an accuracy of 91% on held-out tumor samples and 88% and 83% respectively on independent primary and metastatic samples, roughly double the accuracy of trained pathologists when presented with a metastatic tumour without knowledge of the primary. Surprisingly, adding information on driver mutations reduced accuracy. Our results have clinical applicability, underscore how patterns of somatic passenger mutations encode the state of the cell of origin, and can inform future strategies to detect the source of circulating tumour DNA.
Department/s
- LUCC: Lund University Cancer Centre
- Familial Breast Cancer
- Breastcancer-genetics
- Molecular Cell Biology
- Breast/lungcancer
- Research Group Lung Cancer
Publishing year
2020-02-05
Language
English
Publication/Series
Nature Communications
Volume
11
Document type
Journal article
Publisher
Nature Publishing Group
Topic
- Medical Genetics
Keywords
- Computational Biology/methods
- Deep Learning
- Female
- Genome, Human
- Humans
- Male
- Mutation
- Neoplasm Metastasis
- Neoplasms/genetics
- Reproducibility of Results
- Whole Genome Sequencing
Status
Published
Research group
- Familial Breast Cancer
- Research Group Lung Cancer
ISBN/ISSN/Other
- ISSN: 2041-1723